We are now opening contributions to this blog to start-ups accelerated by our Shake’Up project. Hazy offers a synthetic data generator, combining differential confidentiality, referential integrity, multi-table database support and aerial deployment.
Contingency planning. It’s what the few orgs that are thriving during these multilayered crises have done well.
For those success cases, this planning started at the personnel level. From the CEO and CTO on down, these orgs asked, if a member of the staff gets sick, who is next? What if multiple key players are hospitalized at once? They logged the Internet providers and regions for all on-call engineers and created a chain of replacements if there’s an outage. These orgs made sure not only their internal and customer-facing systems have backups, but that their third-party integration partners did, too.
But some would call all this reacting, not planning. Or simply luck. After all, each organization and industry has its own barriers to overcome. How could any company really prepare for the unknown?
How could any org prepare for a global pandemic if there hasn’t been one of this magnitude for a hundred years?
This is where synthetic data offers an interesting opportunity to hope for the best, but prepare for the worst. Synthetic data — which is highly accurate but highly private, utterly artificial data — can allow your organization to simulate unforeseen events like pandemics and natural disasters.
Synthetic data allows you to contingency plan for even the unpredictable.
What is synthetic data and how is it used?
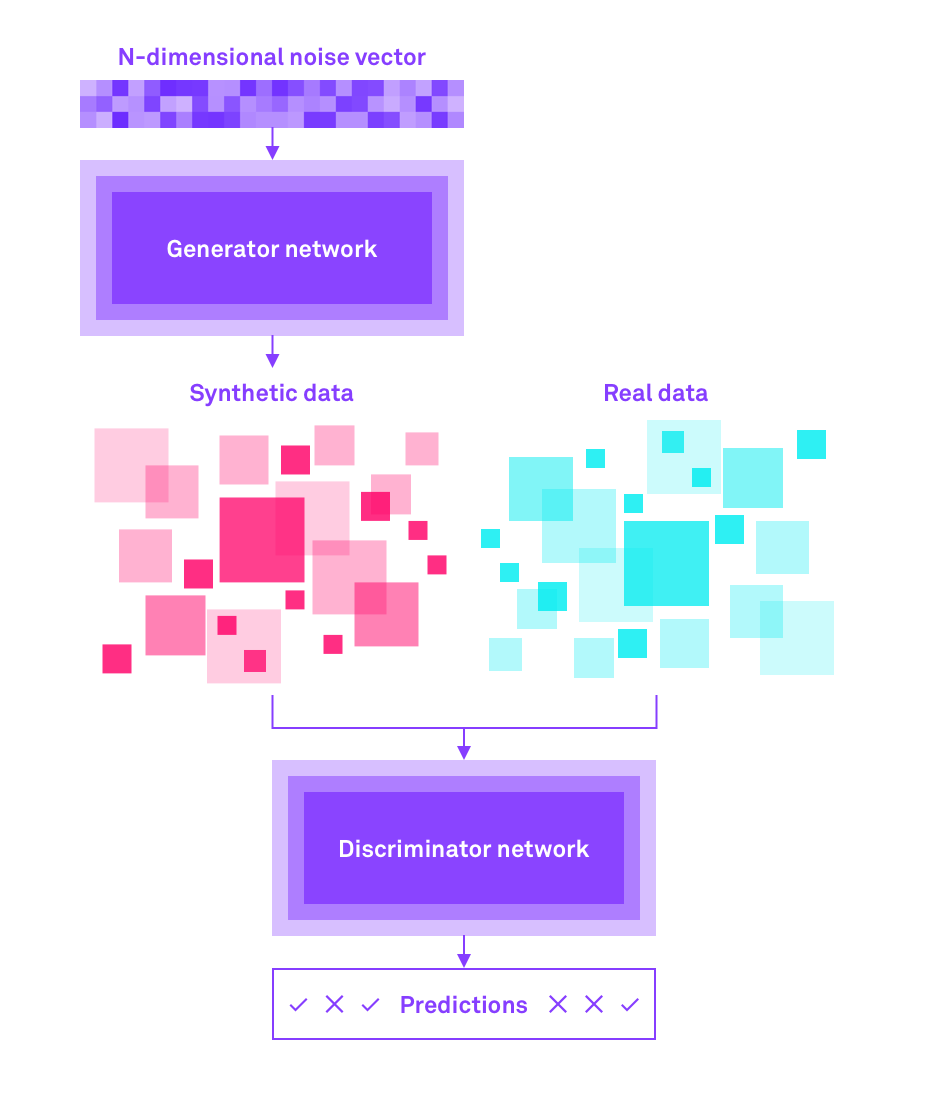
As its name suggests, synthetic data is completely artificial. In the case of Hazy, synthetic data is generated by cutting-edge machine learning algorithms that offer certain mathematical guarantees of both utility and privacy. This is essential because no customer data is really used, while the curves or patterns of their collective profiles and behaviors are preserved.
This is incredibly useful for breaking down barriers to innovation and testing. You can learn all the need-to-know information about your customers, demographics, and habits while dramatically decreasing the risk of re-identification. You can then easily and securely port that synthetic data and insights across different divisions, government agencies, nongovernmental organizations, and geographical restrictions. And you can quickly evaluate third-party integrations partners.
Since smart synthetic data retains both value and compliance, its potential is nearing limitless. It can be applied to solving some of the world’s biggest problems, from escalating international pandemic research and tracing to fairer access to banking to fraud and money laundering detection at a cross-border, cross-organizational scale. It can be used to break down boundaries and optimise cross-governmental collaboration, up until now hindered by divergent databases stuck behind regulatory walls.
Synthetic data allows organizations and governments to overcome both geographical and resource barriers.
Then that synthetic data can even be applied to events that haven’t happened yet.
The world’s leading organizations are starting to leverage synthetic data to build predictive scenarios in order to better respond to future economic, health, political and environmental crises.
It should be noted that synthetic data is not as advanced and mainstream as other enterprise tooling. Since each organization has very complex and varied datasets, they have to be transformed, pre-processed and configured in order to make them accessible to machine learning models. This means while anyone in your org can benefit from synthetic data, your data scientists still have to be involved in this data preparation.
Synthetic data to simulate unforeseen events
Synthetic data is created by generative machine learning models, which, in a way, can be thought of as simulators of the world.
Hazy synthetic data is already being used at major financial institutions for app developers to simulate realistic client behavior patterns before there are even users. This can carry over to machine learning engineers who can better model for this sort of future-demand scenarios.
Our most innovative customers are beginning to extend the use cases of this vanguard technology to these mostly unforeseeable events.
This has only been made a possibility quite recently through conditional synthetic data generation, which allows for the exploration of how some relationships in a dataset can play out with other relationships when their effects are amplified or diminished.
Right now, it’s making headlines in the deep fake images space. Someone could ask a conditional generator for faces that have pink hair, glasses and a nose piercing. Now, the generator may have never seen someone with all of those characteristics combined, but it knows roughly how each of these entities logically combine at a higher level. The machine learning model has learned how lower level entities come together to build meta entities — for example it knows that a nose has a fairly predictable relationship with eyes and mouth. This allows the generator to take what it knows and to accurately fill in the gaps and predict what those punk rockers would look like.
This works slightly differently with customer data like sequential financial data, as these tables often include thousands of columns and have a lot of categorical values — each column can be thought of as a dimension. Working out how categorical values in a table interrelate within a dataset is often more challenging than when working with a dataset consisting of the pixel dimensions of a data set of human faces.
The positive is that banks indisputably have lots of data to work with. Banks also often have access to additional datasets like stock measurements, interest rates, and exchange rates. The interrelationships across different datasets can potentially be combined to better model relationships and explore scenarios and model tradeoffs. With these, machine learning models you can ask questions like how a product might behave when you have a combination like high interest rates and low unemployment.
Maybe the world hasn’t seen that happen in real life, but the generators can be used to extrapolate and fill in the blanks because it generally knows how they trend together.
Insurance companies live in a world of “if then, then this”, but so much of their actuarial insights are based on past data. What can you do if you have no data because these events haven’t happened yet? Synthetic data is a good way to build predictive scenarios that can help organizations adequately price the risk of unforeseen events.
And this crystal ball reading doesn’t have to just be applied to world changing events. You can use synthetic data generators to understand how a new market would react to your launching of a new product.
Say you have a million clients in the UK and only 50,000 in France. And you know the income variability, the geographical zones they live in, and the ages, income and educational level for each customer. First you create synthetic data that protects all the personally identifiable information across two distinct geographic regions. The model then learns both the predictable way the product sold in the UK and it knows the behavioral differences between the two countries. This model can even learn to cleverly extrapolate UK consumer behavior into French consumer behaviour to predict the best way an expansion in the French market might play out. These disparate insights turn into a solid predictor for global expansion KPIs.
These results can again be combined with more probabilities like how your customers or local markets will react depending on how many points the stock market falls or how summer temperatures impact sales. However, if you want to predict very rare events or a combination of rare events with limited data, making predictions remains very challenging without enough data to meaningfully extrapolate trends and relationships in the data.
The limitless potential of securely synthetic data
Synthetic data is the best way to safely unlock the potential of the data economy. Because synthetic data — by being completely artificial — can solve the essential privacy problem, it can significantly reduce data leaks and protect your customers’ personal information, while still retaining utility.
Synthetic data becomes the best way for multinational organizations to stay as competitive, responsive and innovative as startups. And to allow you to capacity plan, based on the completely unknown.
Because large financial institutions have such a wealth of data, they are perfectly positioned to take advantage of the unique potential of data and synthetic data. Organizations can now limit risk-taking by predicting responses for an unpredictable future.
The world is changing rapidly. Your business has to be ready for it.